
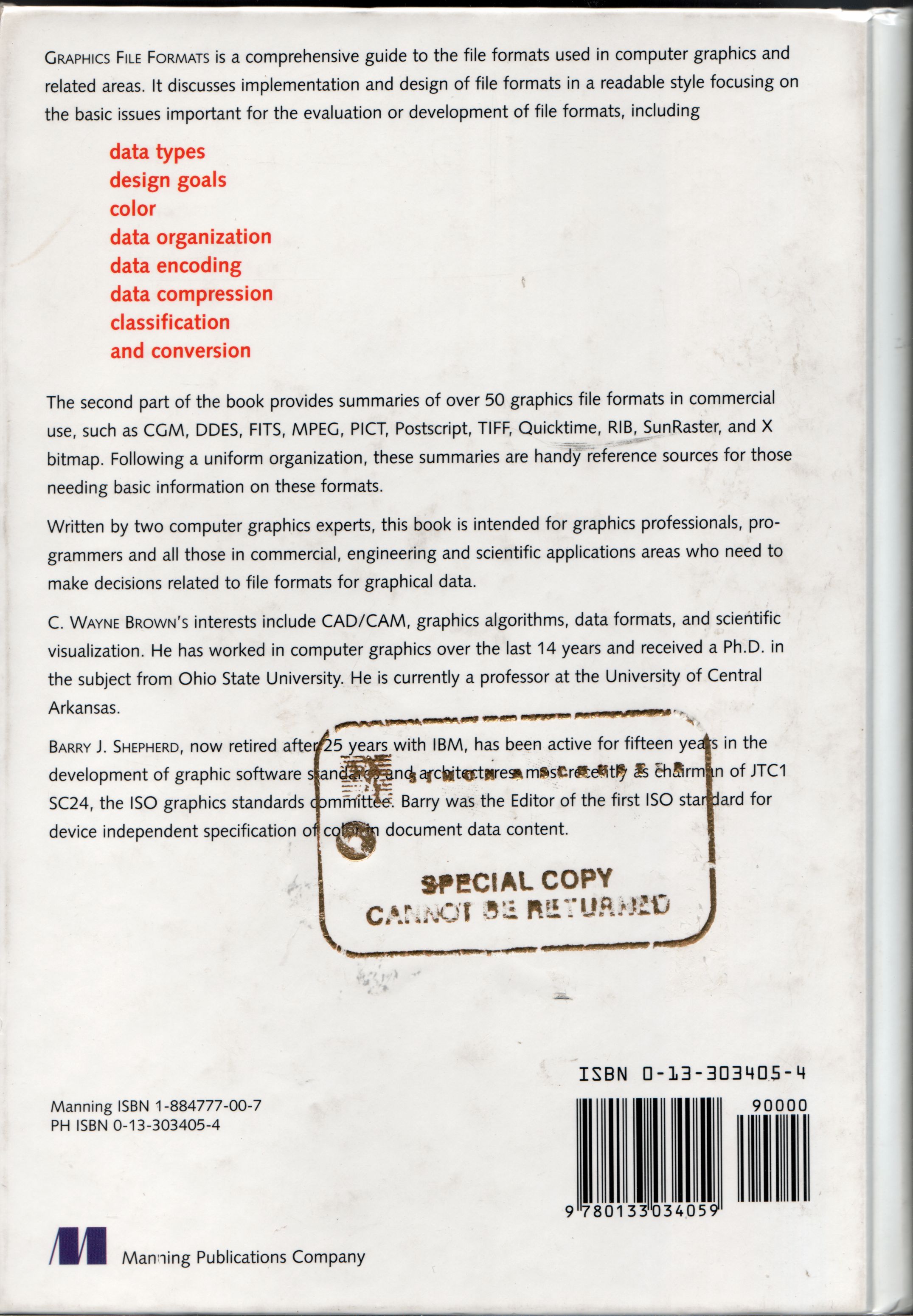
- Cellprofiler analyst exe.log file software#
- Cellprofiler analyst exe.log file code#
- Cellprofiler analyst exe.log file windows#
It began in the late 1970's, and is maintained by an active development community.ĪMPtorch is a PyTorch implementation of the Atomistic Machine-learning Package (AMP) code that seeks to provide users with improved performance and flexibility as compared to the original code. You can also calculate lattice thermal conductivity from first principles.Ī suite of biomolecular simulation programs.
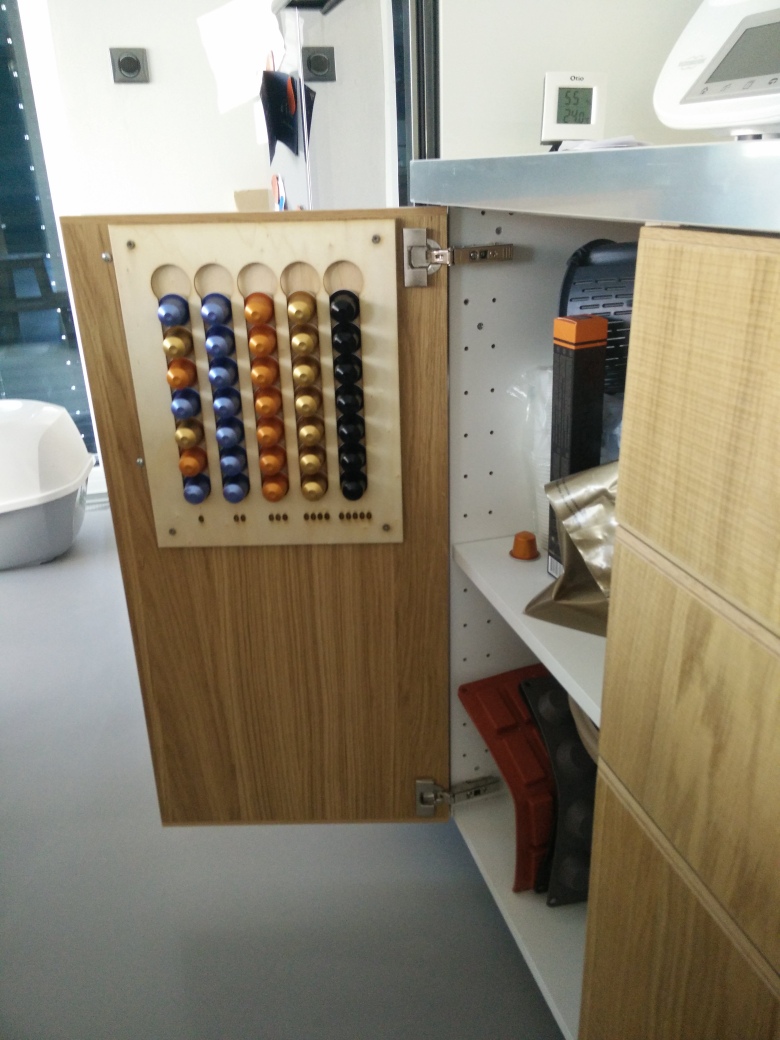
Files are searched for a string or regular expression, with approximate matching capabilities and user-definable records.ĪLAMODE is designed for analyzing lattice anharmonicity and lattice thermal conductivity of solids.īy using an external DFT package such as VASP and Quantum ESPRESSO, you can extract harmonic andĪnharmonic force constants straightforwardly with ALAMODE.
Cellprofiler analyst exe.log file windows#
Binary Packages are provided for MacOS and Linux systems including Fedora, Ubuntu (including Ubuntu under the Windows Subsytem for Linux)ĪGREP - approximate GREP for fast fuzzy string searching.
Cellprofiler analyst exe.log file software#
The software is made to run on virtually an Unix system with X11 and Motif displays. It is freely available (both in source code and in precompiled binaries) for research purposes. Theory (DFT), using pseudopotentials and a planewave or wavelet basis.ĪFNI (Analysis of Functional NeuroImages) is a leading software suite of C, Python, R programs and shell scripts primarily developed for the analysis and display of anatomical and functional MRI (FMRI) data. Third, students of data science who want to understand both the theoretical and practical aspects of data science, vetted by real-world case studies at the intersection of academia and industry.ABINIT is a package whose main program allows one to find the total energy, charge density andĮlectronic structure of systems made of electrons and nuclei (molecules and periodic solids) within Density Functional Second, decision makers in businesses who face the challenge of creating or implementing a data-driven strategy and who want to learn from success stories spanning a range of industries. The book targets professionals as well as students of data science: first, practicing data scientists in industry and academia who want to broaden their scope and expand their knowledge by drawing on the authors’ combined experience. Moreover, it provides answers to the question of what the mission-critical factors for success in different data science undertakings are. Finally, Part III was again written from the perspective of the editors and summarizes the lessons learned that have been distilled from the case studies in Part II. The section can be viewed as a meta-study on data science across a broad range of domains, viewpoints and fields. The case studies presented, and the methods and tools applied, represent the nuts and bolts of data science. Part II then broadens the spectrum by presenting views and insights from diverse authors – some from academia and some from industry, ranging from financial to health and from manufacturing to e-commerce. Each of these chapters describes a fundamental principle, method or tool in data science by analyzing specific use cases and drawing concrete conclusions from them. These six chapters are geared towards drawing a consistent picture of data science and were predominantly written by the editors themselves. With these goals in mind, the book is divided into three parts: Part I pays tribute to the interdisciplinary nature of data science and provides a common understanding of data science terminology for readers with different backgrounds. As such, it is not a replacement for a classical textbook (i.e., it does not elaborate on fundamentals of methods and principles described elsewhere), but systematically highlights the connection between theory, on the one hand, and its application in specific use cases, on the other. This book has two main goals: to define data science through the work of data scientists and their results, namely data products, while simultaneously providing the reader with relevant lessons learned from applied data science projects at the intersection of academia and industry.
